Cursor Team: Future of Programming with AI | Lex Fridman Podcast #447
null
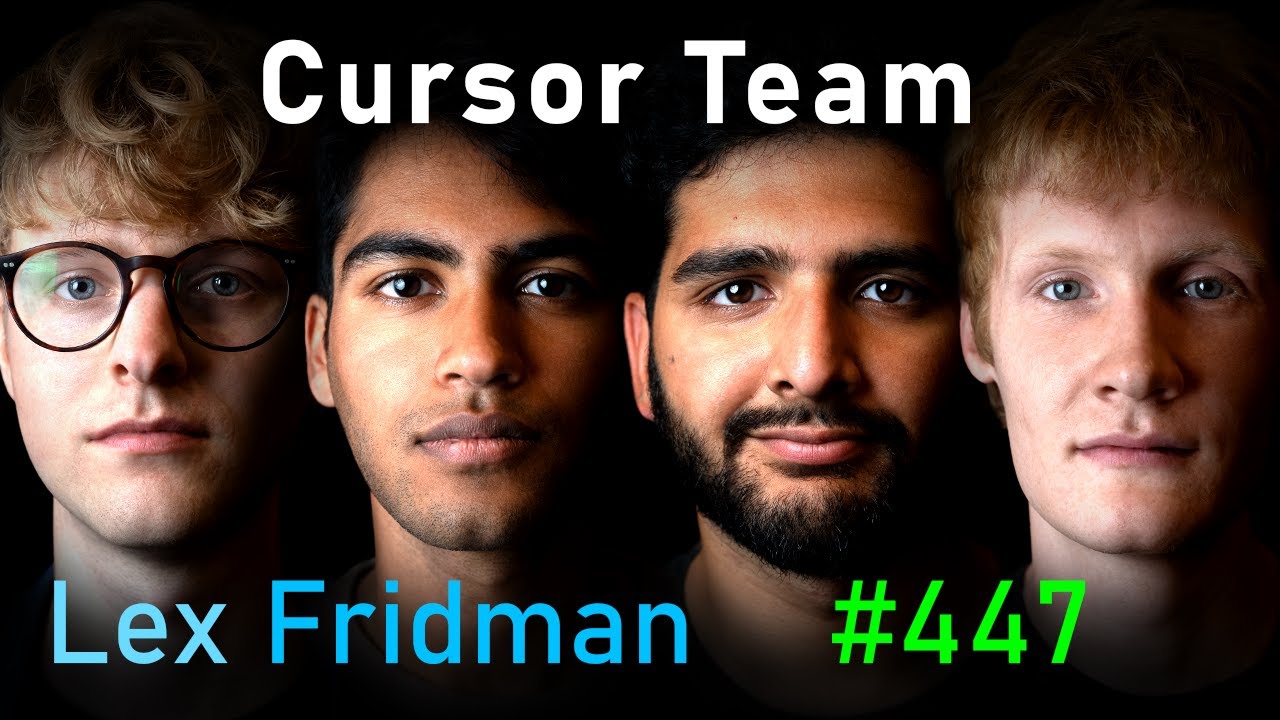
Click
Use
to move to a smaller summary and to move to a larger one
The Role and Future of AI in Code Editing - A Conversation with the Founding Members of the Cursor Team
- A code editor is a powerful tool for building software, providing structure and functionality for editing programming language.
- Code editors offer features like visual differentiation of code, navigation within the codebase, error checking, and more.
- The concept of code editors is likely to evolve in the next 10 years as the process of software development changes.
- Building a code editor that is fun and fast is a priority for the Cursor team.
- The team started out as Vim users but switched to VS Code due to the availability of Co-Pilot, an AI-assisted coding tool.
- Co-Pilot's integration with VS Code inspired the team to create Cursor, a fork of VS Code with additional AI-powered features.
Introduction to Copala and Cursor
- Copala is an autocomplete tool that suggests lines to complete as the user types.
- It aims to provide a smooth and intuitive user experience, similar to when a close friend completes your sentences.
- Even when Copala is wrong, it is not overly frustrating as the user can easily iterate and fix it.
- Copala is considered the first real AI product and a killer app for LMS (language model consumer product).
- Cursor is a project that came about after the release of the scaling loss papers in 2020.
- The papers suggested that bigger model sizes and more data could greatly improve machine learning.
- The theoretical gains predicted in the papers became concrete when early access to gbd4 was obtained in 2022.
- The capabilities of gbd4 sparked the idea that a lot more could be built using AI technology.
- Prior to Cursor, the team had been working on specific tools for different professions, such as financial professionals and static analysis.
- The Step Up in capabilities of gbd4 made it clear that the predicted gains could be realized and led to the development of Cursor.
Building a New Programming Environment and the Decision to Fork VS Code
- Wanted to create a programming environment where all of programming could flow through AI models.
- Felt that this required a different type of programming environment.
- Had a bet with a roommate about winning a gold medal in the International Math Olympiad (IMO) using AI models.
- Initially doubted the possibility of winning, but later realized the potential of scaling laws and became optimistic about progress.
- Started to rethink how AI could be integrated into the editing process and decided to Fork VS Code.
- Chose to Fork VS Code due to its popularity and the need to overcome limitations for better AI integration.
- The decision was made considering the increasing capabilities of AI models and the desire to build a comprehensive AI-powered editor.
The Competitive Advantage of Cursor in the AI Programming Space.
- Cursor aims to improve the capabilities of AI programming.
- Cursor's goal is to change how software is built, leading to productivity gains and radical changes.
- Cursor avoids limitations by not being a plugin to an existing coding environment.
- Cursor focuses on building the most useful features.
- Being ahead in AI programming makes a product more useful.
- Startup companies, like Cursor, have an advantage in innovating and pushing the boundaries.
- Cursor's focus is on capabilities for programmers, rather than just adding features.
- Cursor aims to implement new ideas and improve the overall coding experience.
- Cursor addresses frustrations with the lack of new features in existing tools.
- Cursor's all-in-one approach, with the same people working on UX and model improvement, helps create a better overall experience.
Features of the "tab" function in the all-knowing praise B AI.
- Tab function acts as a fast colleague that predicts and types what the user is going to do next.
- It goes beyond predicting characters and can even predict the next entire change or jump in code.
- It helps users instruct the AI and transition from instructions to code.
- The editing experience for both predicting and instructing is made ergonomic, smart, and fast.
- The model has the ability to edit code and multiple attempts were made to improve this feature.
- Efforts were made to make the inference fast and provide a good user experience.
- The tab function allows users to easily jump to different places in the code after accepting an edit.
- The goal is to make it obvious where to go next after making a change, so the user can simply press tab.
- The internal competition was to determine how many tabs the user needs to press to achieve their desired outcome.
- The idea is to eliminate low entropy actions and make the model read the user's mind to predict their intent.
Details of Next Cursor Prediction and its Implementation
- Next cursor prediction is a task that requires low latency and small models trained specifically for this task.
- It utilizes a sparse model to efficiently handle long prompts with minimal token generation.
- The implementation includes a variant of speculative decoding called speculative edits, which improves performance and quality.
- Caching plays a crucial role in reducing latency and GPU load by reusing the KV cache across requests.
- The near-term goals of next cursor prediction include generating code, filling empty space, editing code across multiple lines, jumping to different locations within the same file, and potentially jumping to different files.
- The ultimate goal is to provide next action predictions, such as suggesting terminal commands based on the written code and navigating to relevant definitions to verify completion suggestions.
- Integration with external services, like Prime Jen's coffee ordering system via SSH, is possible to enhance the user experience.
- The ideal outcome is a system that can predict the next few minutes of a programmer's task based on recent actions, allowing for seamless navigation and efficient coding. The interface also includes a diff display for better understanding of changes.
Proposed Modifications to Diff Interface for Code Review
- The current diff interface for code review has a box on the side that shows deleted and added code, which can be distracting.
- Different attempts have been made to improve the interface, including using blue crossed out lines and red highlighting.
- Another iteration involved using blue highlighting on code regions to indicate AI suggestions, which can be viewed by holding the option button.
- The current interface is not intuitive and may require improvements.
- Proposed improvements include highlighting important parts of the diff and graying out less important sections, as well as using a model to identify potential bugs and mark them for review.
Improving Code Review and Assistance with Language Models
- Language models can be used to improve code review by guiding reviewers through code sections that matter and pointing out regions of interest.
- Code review can be time-consuming and often doesn't catch many bugs, but language models can significantly enhance the review experience.
- By designing the code review process around the reviewer and not the code producer, the focus can be on making the reviewer's job more enjoyable, easy, and productive.
- Ordering matters in code review, and language models can assist by guiding reviewers through code sections in a logical sequence.
- Not all programming will be done in natural language, as sometimes it may be easier to communicate with examples or through visual interfaces.
- Natural language, examples, visual interfaces, and future technologies like brain-machine interfaces may all play a role in communicating with AI in programming.
ML Models and Making Cursor Tab Fast
- Cursor Tab uses an ensemble of custom models and frontier models.
- Apply model is used to create code changes based on the sketches provided by the frontier models.
- Deterministic algorithms for combining sketches and applying changes often fail, resulting in a poor user experience.
- Using fewer tokens with intelligent models like Apply improves latency and cost.
- Future models could handle higher-level plans recursively while less intelligent models handle implementation details.
- Speculative edits, a variant of speculative decoding, help make Cursor Tab fast.
- Speculative decoding processes multiple tokens at once, improving generation speed.
- Strong priors are used instead of small models to predict draft tokens for verification.
Comparison of language models for coding
- Existing code can be fed into the model to generate similar code.
- The model can process multiple lines of code in parallel.
- The model reaches a point of disagreement with the original code and generates different tokens.
- This approach allows for faster editing of code compared to traditional methods.
- Wireless streaming allows for code review before completion, eliminating the need for a loading screen.
- Speculation is a common idea in various fields, including language models and CPUs.
- Sonnet is currently considered the best model for coding, with good reasoning abilities.
- Other models perform well on benchmarks but struggle outside of those contexts.
- Benchmarks may not accurately represent real coding scenarios, which are more context-dependent and involve instructions in broken English and varied requirements.
Challenges in Understanding and Meeting Human Expectations in AI Models
- Interview problems are well-specified, while human understanding is less specified.
- Difficulty in modeling real-world programming due to lack of clear specifications.
- Public benchmarks can be contaminated and hard to obtain accurate data from.
- Training models on popular repositories may not provide true evaluation scores.
- Qualitative feedback and human assessment are used to gauge model performance.
- The need for "vibe checks" and subjective opinions to evaluate model effectiveness.
- Differences in hardware and numerical computations may affect model performance.
- Some speculate AWS chips may have affected the performance of the model.
Importance of Prompt Design in Maximizing Success
- Good prompt design plays a crucial role in maximizing success.
- Benchmark models respond differently to different prompts.
- Original models like gp4 and bre were sensitive to prompts and had a small context window.
- Deciding what to include in a prompt can be challenging due to limited space.
- Filling out the entire context window can slow down the model and cause confusion.
- Preum, a system internally used by OpenAI, helps with prompt design by managing the context window.
- Prompt design is similar to designing a website, where the content needs to be formatted to fit different devices.
- OpenAI takes inspiration from the declarative approach of React in prompt design.
- Prompt design involves declaring what is needed and letting the rendering engine fit everything onto the page.
- React-like components are used in prompt design, such as a file component that considers line priorities for rendering.
- Prompt design helps with data splitting and debugging, allowing for changes to be tested on old prompts.
- JSX is used for prompting, making it resemble React components.
Improving User Experience and Addressing Uncertainty in Coding with Agents and Retrieval Techniques
- Code blocks can be retrieved and prioritized using techniques such as embedding and reranking scores.
- The goal is to allow users to write code in the most natural and intuitive way, while ensuring that the system can understand their intent.
- There is a tension between allowing users to be lazy and providing more prompts to encourage articulate problem descriptions.
- When users don't convey enough intent, the system can ask for clarification or present multiple options for the user to choose from.
- Suggestions for additional files or edits can be made based on the current prompt and previous commits, but accuracy is still being improved.
- Agents are seen as promising for improving the coding experience, resembling human-like behavior and bringing us closer to AGI.
- However, agents are not yet widely useful, but there are certain tasks where having an agent would be beneficial.
Bug with copy-pasting in chat input box and the potential for programming agents in Cursor.
- Bug where users can't use copy and paste in the chat input box.
- Request for an agent that can fix the bug by reproducing, fixing, and verifying it.
- Mention of the belief that agents will take over all of programming, but acknowledgment that iteration is important in programming.
- Interest in having an agent that can instantly provide an initial version for quick iteration.
- Discussion about an agent that can set up the development environment, install software packages, configure databases, and deploy the app.
- Clarification that Cursor is not actively working on this feature but wants to make programming easier and more enjoyable.
- Idea of having a background agent that can work on certain tasks while the user focuses on other aspects.
- Emphasis on the need for speed in Cursor and mention of the slowest aspect being the apply function, which is being worked on.
- Use of caching as a strategy to improve speed, such as prefilling the cache with file contents as the user types.
- Mention of lower latency and cost with cross requests.
Explanation of KV Cache and the benefits of caching in Transformers.
- Transformers use keys and values to enable attention and allow them to consider previous tokens.
- By storing keys and values of previous tokens, the model can avoid performing a forward pass through the entire model for every token, significantly reducing the time to compute.
- Caching prompts or using higher-level caching techniques, such as speculative caching, can further improve performance.
- Speculative caching involves predicting ahead as if the user had accepted a suggestion, allowing for faster retrieval of the next suggestion when the user presses "tab".
- Smaller KV caches can enable more speculation and improve the chances of predicting the user's intended input.
- RL (Reinforcement Learning) exploits the phenomenon of predicting multiple options to increase the chances of predicting the correct one.
- RL in cursor Tab models predicts which suggestion is more desirable to humans out of many different suggestions.
- The model internally has some uncertainty about which key information is correct or preferred by humans.
- Caching allows the model to make predictions farther ahead, potentially improving accuracy and relevance of suggestions.
Techniques to Improve Model Performance and Speed
- Reward things that humans prefer and punish things they dislike.
- Train the model to generate suggestions that humans prefer using RL loops.
- Efficient attention schemes like multiquery attention and group query attention help generate tokens faster.
- Compressing the size of key and value caches improves memory bandwidth and speeds up token generation.
- Techniques like multi- latent algorithm (MLA) reduce the number of key-value heads while preserving diversity.
- MLA utilizes a shared vector for keys and values, along with smaller vectors for individual tokens, and expands them efficiently during computation.
- Low rank reduction can be used to store smaller vectors and expand them later for more efficient memory usage.
Benefits of Using a Larger KV Cache and Background Computation in a User Experience
- Larger KV cache allows for more aggressive caching and increased cache hits, resulting in reduced time to First token.
- Background computation enables faster generation of tokens during inference with larger batch sizes, without experiencing significant slowdown.
- Increased size of prompts or batch sizes can be accommodated without degrading latency.
- Shadow workspace allows for computation in the background, providing long-term predictions for the user.
- Language server protocol, used in Cursor, enhances the coding experience by offering features like linting, type checking, and going to definition.
- Language server protocol is also integrated with the models in Cursor, allowing them to access and utilize the same information as the programmer.
- This integration helps in providing relevant feedback and improving the performance of the models.
Creating a Shadow Workspace for Background Coding
- The idea behind the Shadow Workspace is to have a separate hidden window where AI agents can modify code without saving it.
- The AI agents can receive feedback from filters and go to definition to iterate on their code.
- The code is run and modified in the background, as if it is in the user's environment.
- On Linux, the file system can be mirrored, allowing the AI to make changes to the files stored in memory.
- On Mac and Windows, holding a lock on saving can be implemented, allowing changes to be made to the shadow workspace instead of the ground truth version of the files.
- Allowing AI models to change files is an exciting feature that can be compared to working with a colleague.
- For simple tasks performed in a few minutes, working locally on the user's machine is sufficient.
- For more complex tasks that take longer periods of time, a remote sandbox environment may be necessary.
- Agents for coding can include bug finders and feature implementers.
- Agents can also be used for tasks like video editing, automation, translation, and overdubbing.
Challenges with Bug Finding in AI Models
- AI models struggle with bug finding due to poor calibration and limited examples.
- Pre-training with large amounts of code data helps models excel at code generation and question answering tasks.
- Models face difficulties with tasks that have limited online presence, such as bug detection and proposing fixes.
- Transferring models from pre-trained code representations to bug detection requires nudging in the right direction.
- Models may understand code well during pre-training but struggle to identify the importance and severity of bugs.
- Human calibration of bug importance plays a crucial role in bug detection.
- Determining which lines of code are critical and require attention is a challenge for both models and humans.
Importance of Labeling Code and the Potential for Future Formal Verification
- Labeling code with warnings and reminders is important to prevent mistakes and potential damage.
- Labeling code is beneficial for AI models as it helps them pay more attention to potential issues.
- Some people find labeling code to be aesthetically unpleasing, but it is useful for preventing errors.
- Labeling code is necessary because humans often forget important details and can easily make small mistakes.
- Formal verification could potentially eliminate the need for extensive testing and allow for the automatic generation of code specifications.
- However, formal verification may be challenging due to the difficulty of specifying intent and generating accurate specifications.
- Spec languages may need to evolve to capture more nuanced aspects of code behavior.
- The ultimate goal is to have formal verification for entire code bases, although this is more challenging than for individual functions.
Bug Finding and Verification in Code and AI
- The process of formally verifying code can be done at multiple layers, from C code to the hardware level.
- Decomposing a big code base and verifying each part individually is possible.
- Handling side effects and external dependencies, such as calling the stripe API, can be challenging.
- Language models used as primitives in programs may introduce dependencies that need to be considered during verification.
- Proving the alignment and correctness of language models is a potential goal.
- Bug finding models can help catch common and complex bugs, making code more reliable.
- Verifying AI-generated code becomes crucial as AI takes on more programming tasks.
- One approach to training bug finding models involves introducing synthetic bugs and training a reverse bug model.
- Providing additional information to models, such as traces and debugger access, can improve bug finding capabilities.
- Different product form factors may emerge in bug finding and verification tools for code and AI.
Integration of Money and Bug Bounties in the Coding Assistant
- Consideration of integrating money into the coding assistant for bug finding and code generation.
- Mention of willingness to pay a significant amount of money for bug finding or generating appreciated code.
- Discussion on the potential benefits and drawbacks of introducing a bug bounty system.
- Controversial idea within the company, with considerations of trust in humanity and impact on the user experience.
- Suggestion of a tipping component or a separate fee system for accessing additional features.
- Potential concern about users copying and pasting code without putting in effort to find bugs.
- Mention of the need for a technical solution to verify fixed bugs and reduce reliance on the honor system.
- Question about the extent of interaction between the terminal and the code, and if the coding assistant can suggest changes based on runtime errors.
Challenges and Infrastructure Choices in Scaling a Startup
- Separate worlds exist within the system, including terminal controls and database operations.
- Looping functionality is still being developed and considered for implementation.
- Question arises whether operations should occur in the foreground or background.
- New API being developed, such as the ability to add branches to a database, to facilitate feature testing without modifying the prod database.
- Technical complexity involved in correctly implementing branching in the write-ahead log of a database.
- Turbo buffer, one of the used databases, may introduce branching support in the future.
- Branching may become a requirement for databases to support AI agents and their testing processes.
- Idea of branching extended to file systems is intriguing and could be beneficial.
- Branching presents challenges in terms of space and CPU usage, but clever algorithms can help mitigate these.
- AWS is chosen as the primary infrastructure provider due to its reliability and trustworthiness.
- AWS products are known to work well, even though the setup process can be challenging and the interface may be lacking.
- Scaling to accommodate a large number of users brings various challenges, such as encountering issues with caching and databases.
- Adding extra zeros to the request per second exposes scalability issues, including integer overflows in tables.
- Custom systems, like the codebase semantic indexing and answering questions, have proven to be particularly tricky to scale.
Technical Challenges and Solutions for Codebase Indexing
- Code embeddings are uploaded and stored in a database, while actual code is not stored.
- Ensuring client-server synchronization is a technical challenge.
- Hashes are used to reconcile the state between the client and the server.
- Reconciling the root hash of a project is the primary focus to minimize network and database overhead.
- Hierarchical reconciliation is performed if there are hash mismatches.
- The Merkel tree is utilized for hierarchical reconciliation.
- Scaling the codebase indexing system for a large number of users and large codebases is a difficult problem.
- Clever ideas and ongoing development are being implemented to improve scaling and efficiency.
Benefits of Indexing and Retrieving Code Base
- Faster code retrieval for individuals accessing the code base.
- Code vectors stored instead of the entire code, reducing storage requirements.
- Improved searching capabilities for locating specific code sections.
- Potential for future enhancements in retrieval quality.
- Local embedding models are difficult to implement due to hardware limitations and resource-intensive processes.
- Processing large code bases locally can be challenging, even on powerful computers.
- Local models may not be feasible for companies with extensive code bases.
- Cloud-based indexing and retrieval provide a better experience for users.
Challenges with Local Models and the Potential of Homomorphic Encryption for Privacy-Preserving Machine Learning
- Using local models can consume excessive memory and CPU resources.
- Models are becoming larger and may not fit on a single node, requiring multiple nodes.
- Local models may not be able to match the capabilities of more powerful cloud-based models.
- There is a resistance against relying on centralized power centers and a preference for local models in the open source movement.
- Homomorphic encryption for language model inference could provide an alternative to local models, allowing encrypted data to be processed on servers without revealing the data itself.
- Research on homomorphic encryption is still ongoing, with the aim of reducing the overhead.
- Centralized control of data flowing through a few actors raises concerns about surveillance and misuse of information.
- Privacy-preserving machine learning is a challenge, but it could help mitigate the downsides of relying on cloud-based models and centralized data control.
Concerns about Centralization and Control in AI Models
- Worries about the entropic scaling policy and centralized monitoring of prompts.
- Concerns about the heavy monitoring and centralization of all the world's information.
- Comparing the difference between AI model providers and cloud providers in terms of data collection.
- The challenge of automatically determining the context for AI models.
- Trade-offs in including automatic context, such as slower performance and higher costs.
- Ideas for improving automatic context computation and retrieval systems.
- Exploring the possibility of language models understanding new information and infinite context.
- Trying different approaches, such as fine-tuning at the weight level or the context learning level.
Improving Retrieval Systems and Training Models to Understand Code Bases
- US as a company is excited about better retrieval systems and picking relevant parts of the code base.
- One interesting proof of concept involves training models directly in weights using VS Code as an example.
- Models are fine-tuned to answer questions about code in general, but there is potential to specifically train models to understand a specific code base.
- There is uncertainty about whether the retrieval should be done within the model or if it should be separated.
- Post-training a model to understand a code base involves replicating the pre-training process with specific code data and fine-tuning with code-related questions.
- Synthetic data or ground truth data can be used in this process to enhance the model's ability to answer questions about the code base.
- Test time compute is an interesting approach to scale up performance when training models, especially as scaling up the amount of data becomes challenging.
Maximizing Model Efficiency and Intelligence for Different Query Types
- Training a bigger model with more flops is not always necessary for all queries.
- A smaller model trained for longer can achieve similar quality as a larger model.
- Only a small percentage of queries require a 100 trillion parameter model.
- It is inefficient to spend excessive compute on training and running a model for infrequent queries.
- Dynamic model routing is a research problem and no solution has been found yet.
- Determining the required level of intelligence for different problems is challenging.
- Test time compute requires a specific training strategy.
- Understanding the inner workings of models like GPT-4 is not clear outside of big labs like OpenAI.
- The use of reward models, such as process reward models, could be beneficial for competing models.
- Process reward models grade the chain of thought rather than just the final outcome.
- OpenAI has experimented with human labelers to create a dataset for process reward models.
- Current utilization of process reward models is limited to sample selection.
Training Process Reward Models and Hiding the Chain of Thought
- Papers sample outputs from language models and use process reward models to grade them.
- The use of process reward models in tree search is an interesting area of research.
- Training process reward models in a more automated way is an ongoing focus.
- Open AI's decision to hide the Chain of Thought from users may be to protect the technology from being replicated.
- Access to log probabilities can provide valuable information for distilling capabilities from APIs.
- Open AI is still exploring how to integrate the GPT-3 model into an editor effectively.
- The potential use cases for GPT-3 are still being discovered.
Limitations and Future of a Product, Integration of Co-Pilot, and the Value of Innovation
- The product has significant limitations, such as the lack of streaming capabilities and a cumbersome editing experience.
- The current version of the product feels like an early stage of test time computing search, with many areas for improvement.
- Integration of GitHub's Co-Pilot with the product might lead some to believe that the existing product, Cursor, should be shut down.
- The software space in which the product operates has a high potential for growth and improvement.
- Building the best product and continuously innovating is essential for success in this market.
- Startups have an opportunity to enter the market by creating something better than existing products.
- The value of the product lies not only in integrating new models quickly but also in the depth and thoughtful user experience.
- Synthetic data can be categorized into three main types: distillation, where a language model outputs tokens or probability distributions for training less capable models; problems where introducing bugs is easier than detecting them; and data generation through simulation or procedural methods.
Approaches to Synthetic Data and Verification in AI Models
- Using synthetic data to train models for bug detection.
- Producing texts with language models that can be easily verified.
- Training models with OKAY model-generated rollouts that prove ground truth theorems.
- Verifying code by testing and training models on passing outputs.
- Challenges in finding the perfect verifier for open-ended tasks.
- RL with feedback (rhf) involves training reward models using human feedback.
- RHF works if sufficient human feedback can be obtained.
- RL with feedback (rif) can be effective if verification is easier than generation.
- RIF may involve recursively improving language model outputs through verification.
- A mix of RIF and RHF can be used, where models are mostly correct and require some human guidance.
- Comparing generation and verification in terms of their performance and intuition.
Scaling Laws and Optimization Strategies in AI
- The original scaling laws paper by OpenAI had some issues with learning rate schedules.
- Chinchilla showed a more correct version of the scaling laws.
- People have deviated from optimizing for the computer optimal thing and started optimizing for making the system work well given an inference budget.
- There are more dimensions to the scaling curves than just compute, parameters, and data.
- Context length is an important factor in scaling, along with inference compute.
- Training models like SSMs may be more suitable for long context windows, despite requiring more compute during training.
- Optimization strategies may prioritize certain factors based on specific use cases and goals.
Investing in Computational Resources for Maximizing Raw Intelligence
- The original conception was to measure the size of the model and data using parameters and tokens, and look at the ratio between them.
- Bigger models generally lead to better raw performance and intelligence.
- Distillation is a promising approach to achieve a more capable and faster model by training on a large model and then distilling the knowledge into a smaller one.
- Distillation helps overcome the limitation of limited training data by extracting more signal per token.
- If given a large amount of money, it would be important to have access to the secrets and details known only to large labs to avoid wasting the resources.
- Investing in computational resources, specifically GPUs, would be crucial for maximizing raw intelligence.
- Increasing compute power allows for running more experiments and tuning between big and small models.
- However, it is also important to consider other limitations such as availability of compute and the need for expertise in utilizing resources effectively.
The Future of Programming and Research in AI
- Limitations in AI research are not just due to compute power and data, but also to the scarcity of talented engineers who can effectively implement ideas.
- The engineering effort involved in transforming research concepts into working models is extensive and requires exceptional skills.
- Reducing the cost and complexity of engineering would significantly accelerate research progress.
- Low hanging fruit, like scaling existing models, should be pursued first before exploring new ideas.
- With massive investment, reevaluating ideas and exploring new ones becomes essential.
- Testing new ideas on smaller scales is possible, but current labs have limited resources to dedicate to such explorations.
- The nature of programming is expected to change in the future, emphasizing speed, agency, and control for programmers.
- The ability to modify anything and iterate quickly will be key in the programming landscape.
The Importance of Human Control and Speed in Software Engineering
- Talking to computers and having them build software is an idea that lacks specificity and control.
- Engineering involves making important decisions and trade-offs, which should be dictated by humans.
- The concept of controlling the level of abstraction in a codebase and editing pseudo code could provide productivity gains while still keeping human control.
- The balance between control, speed, and human involvement in programming is crucial.
- The skill of programming is evolving, and despite concerns, it is an exciting time to be in the field.
The Future of Programming and the Changing Landscape
- Programming is becoming more focused on delight, speed, and individual control.
- The skills and creative ideas of programmers will be amplified, making it a more enjoyable experience.
- AI tools will make coding faster and easier, allowing for rapid iterations and less upfront planning.
- Generating boilerplate code will be automated, freeing up time to focus on more complex design decisions.
- The fear is that as AI models improve, there may be less room for creative decision-making and programming may become more like natural language processing.
- JavaScript is predicted to be the dominant programming language in the future.
- The traditional idea of who can be a programmer is expanding, and a broader range of individuals can excel in programming.
The Passion of Programmers and the Future of Programming
- The best programmers are the ones who have a true love and obsession for programming.
- Some programmers dedicate their free time to coding on side projects late into the night.
- The love of programming drives these individuals to get into the details of how things work.
- Pressing the tab key is more than just a simple action; it involves injecting intent and shaping what is being created.
- Programming is evolving towards higher bandwidth communication with computers, where intent is communicated more effectively.
- There is an ongoing effort to build a hybrid human-AI programmer that is more effective and efficient than any one engineer.
- This hybrid engineer will have effortless control over their code base and iterate at the speed of their judgment.
- The goal is to outsmart and out-engineer pure AI systems.
- The work of researchers and engineers in this field has already improved the lives of hundreds of thousands of programmers.
- The aim is to make programming more fun and enjoyable for everyone.
Overview of Cursor, an AI-powered code editor
- Cursor is a fork of VS Code with additional AI-powered features.
- It aims to provide a smooth and intuitive user experience with features like Co-Pilot and Copala.
- Cursor was developed after the release of scaling loss papers and the availability of gbd4.
- The goal of Cursor is to create a programming environment where all programming can flow through AI models.
- It focuses on improving AI capabilities in programming and aims to change how software is built.
- Cursor avoids limitations by not being a plugin and focuses on building the most useful features.
- It addresses frustrations with existing tools and aims to provide a comprehensive AI-powered editor.
- Tab function acts as a fast colleague, predicting and typing what the user is going to do next.
- The editing experience for both predicting and instructing is made ergonomic, smart, and fast.
- The goal is to make it obvious where to go next after making a change, eliminating low entropy actions.
Next Cursor Prediction and Code Review Improvements
- Next cursor prediction:
- Requires low latency and small models trained specifically for the task.
- Utilizes a sparse model for efficient handling of long prompts.
- Implements speculative decoding called speculative edits for improved performance and quality.
- Utilizes caching to reduce latency and GPU load.
- Goals include generating code, filling empty space, editing code across multiple lines, and jumping within files.
- Integration with external services, like Prime Jen's coffee ordering system via SSH, is possible.
- Aims to provide next action predictions and enhance user experience in coding.
- Diff interface for code review:
- Current interface includes a box on the side showing deleted and added code, which can be distracting.
- Proposed improvements include highlighting important parts and graying out less important sections.
- Using a model to identify potential bugs and mark them for review.
- Language models for code review:
- Language models can guide reviewers through code sections and point out regions of interest.
- Can significantly enhance the code review experience.
- Designing the code review process around the reviewer can make the job more enjoyable and productive.
- Language models can assist by guiding reviewers through code sections in a logical sequence.
- Communication with AI in programming:
- Natural language, examples, visual interfaces, and future technologies like brain-machine interfaces can play a role.
- Not all programming will be done in natural language.
- Cursor Tab uses an ensemble of custom and frontier models for code generation.
- Speculative edits and Cursor Tab:
Maximizing Success with Prompt Design and Improving Cursor Speed
- Benchmark models respond differently to prompts.
- Prompt design is similar to designing a website.
- React-like components are used in prompt design.
- Prompt design helps with data splitting and debugging.
- JSX is used for prompting.
- Code blocks can be retrieved and prioritized.
- The goal is to allow users to write code naturally.
- Suggestions for additional files or edits can be made.
- Agents are seen as promising for improving the coding experience.
- Bug where users can't use copy and paste in the chat input box.
- Request for an agent that can fix the bug.
- Interest in having an agent that can instantly provide an initial version.
- Discussion about an agent that can set up the development environment.
- Clarification that Cursor is not actively working on this feature.
- Idea of having a background agent that can work on certain tasks.
- Emphasis on the need for speed in Cursor.
- Use of caching as a strategy to improve speed.
- Transformers use keys and values to enable attention.
- Caching prompts or using higher-level caching techniques.
- Speculative caching involves predicting ahead.
- Smaller KV caches can enable more speculation.
- RL exploits the phenomenon of predicting multiple options.
- Caching allows the model to make predictions farther ahead.
- Efficient attention schemes help generate tokens faster.
- Compressing the size of key and value caches improves speed.
Enhancing Coding Experience with Larger KV Caches, Background Computation, Shadow Workspaces, and Language Server Protocol
- Larger KV cache allows for more aggressive caching, increasing cache hits and reducing time to First token.
- Background computation enables faster token generation during inference with larger batch sizes.
- Increased size of prompts or batch sizes can be accommodated without degrading latency.
- Shadow workspace allows for long-term predictions and computation in the background.
- Language server protocol integrated with Cursor models enhances coding experience with linting, type checking, and going to definition.
- Shadow workspace enables AI agents to modify code without saving it, receiving feedback from filters and going to definition to iterate on their code.
- AI models struggle with bug finding, but pre-training with large amounts of code data helps models excel at code generation and question answering tasks.
- Transferring models from pre-trained code representations to bug detection requires nudging in the right direction.
- Human calibration of bug importance plays a crucial role in bug detection.
- Labeling code with warnings and reminders is important to prevent mistakes and potential damage.
- Formal verification could potentially eliminate extensive testing and allow for automatic code specification generation.
- Handling side effects and external dependencies can be challenging during formal verification.
- Bug finding models can help catch common and complex bugs, making code more reliable.
- Verifying AI-generated code becomes crucial as AI takes on more programming tasks.
- Consideration of integrating money into the coding assistant for bug finding and code generation.
- Discussion on the potential benefits and drawbacks of introducing a bug bounty system.
- Suggestion of a tipping component or separate fee system for accessing additional features.
- Mention of the need for a technical solution to verify fixed bugs and reduce reliance on the honor system.
System Architecture and Scaling Challenges
- Separate worlds exist within the system, including terminal controls and database operations.
- Looping functionality is still being developed and considered for implementation.
- The question arises whether operations should occur in the foreground or background.
- A new API is being developed to add branches to a database for feature testing without modifying the prod database.
- Implementing branching in the write-ahead log of a database is technically complex.
- Turbo buffer, one of the used databases, may introduce branching support in the future.
- Branching may become a requirement for databases to support AI agents and their testing processes.
- The idea of branching extended to file systems is intriguing and could be beneficial.
- Branching presents challenges in terms of space and CPU usage, but clever algorithms can help mitigate these.
- AWS is chosen as the primary infrastructure provider due to its reliability and trustworthiness.
- Scaling to accommodate a large number of users brings challenges such as caching and database issues.
- Custom systems like codebase semantic indexing and answering questions are particularly tricky to scale.
- Code embeddings are stored in a database, while actual code is not stored.
- Reconciling the state between the client and server is a technical challenge using hashes.
- The Merkel tree is utilized for hierarchical reconciliation.
- Scaling the codebase indexing system for a large number of users and codebases is difficult.
- Faster code retrieval is achieved by storing code vectors instead of the entire code.
- Local embedding models are difficult to implement due to hardware limitations and resource-intensive processes.
- Cloud-based indexing and retrieval provide a better experience for users.
- Homomorphic encryption for language model inference could provide an alternative to local models.
Considerations for Training and Utilizing Language Models
- Training a bigger model with more flops is not always necessary for all queries.
- Smaller models trained for longer can achieve similar quality as larger models.
- Only a small percentage of queries require a 100 trillion parameter model.
- Dynamic model routing is a research problem without a solution yet.
- Determining the required level of intelligence for different problems is challenging.
- Test time compute requires a specific training strategy.
- Understanding the inner workings of models like GPT-4 is not clear outside of big labs like OpenAI.
- Reward models, such as process reward models, could benefit competing models.
- OpenAI has experimented with human labelers to create a dataset for process reward models.
- Current utilization of process reward models is limited to sample selection.
- The use of process reward models in tree search is an interesting area of research.
- Training process reward models in a more automated way is an ongoing focus.
- OpenAI's decision to hide the Chain of Thought from users may be to protect the technology from being replicated.
- Access to log probabilities can provide valuable information for distilling capabilities from APIs.
- OpenAI is still exploring how to effectively integrate the GPT-3 model into an editor.
- The potential use cases for GPT-3 are still being discovered.
- The product has limitations such as the lack of streaming capabilities and a cumbersome editing experience.
- The current version of the product feels like an early stage of test time computing search with room for improvement.
- Integration of GitHub's Co-Pilot with the product might lead to the perception that Cursor should be shut down.
- The software space in which the product operates has high potential for growth and improvement.
Limitations and Future of Programming and AI
- Limitations in AI research are not just due to compute power and data, but also the scarcity of talented engineers.
- The engineering effort required to transform research concepts into working models is extensive and requires exceptional skills.
- Lowering the cost and complexity of engineering would accelerate research progress.
- Scaling existing models should be pursued before exploring new ideas.
- Massive investment is necessary to reevaluate and explore new ideas.
- Limited resources in current labs hinder the ability to test new ideas on smaller scales.
- The nature of programming is expected to change, emphasizing speed, agency, and control for programmers.
- The ability to modify anything and iterate quickly will be key in the programming landscape.
- Talking to computers and having them build software lacks specificity and control.
- Programming involves making important decisions and trade-offs that should be dictated by humans.
- Controlling the level of abstraction in a codebase and editing pseudo code could increase productivity while maintaining human control.
- The balance between control, speed, and human involvement in programming is crucial.